June 16, 2022 | AI and NLP, Clinical Data Strategy, Digital Quality Transformation, Year Round Prospective HEDIS®
Data Quality Primer
In this installment of our monthly series, we’ll dig into the problem of data quality. It’s not all doom and gloom. With some planning, you can get the most out of your NLP vendor while paving a path to higher-quality data across your entire organization. This blog will tell you how to do that.
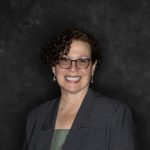
Rebecca Jacobson, MD, MS, FACMI
Co-Founder, CEO, and President
We’ve seen profound innovations in Natural Language Processing (NLP) over the past 15 years, and we are now seeing those innovations crop up in healthcare, in areas like Value-Based Care, Risk Adjustment, and Quality Measurement. If you’ve dabbled in NLP for RA or Quality, you’ve likely found that the benefit you’re looking for (better population management, increased HCC capture, higher quality rates, or improved efficiency) depends on the accuracy of your NLP system. And one thing holding you back might be the quality of your data. Let’s examine why data quality is so important to NLP, and what can go wrong as data quality degrades.
Errors Can Get Magnified
The first thing to understand is that NLP systems mostly work as a sequence of components that process large volumes of data. The first component in the sequence might chop up a document into relevant sections (for example Chief Complaint or History of the Present Illness), while another component down the line identifies clinical terms of interest (for example Colonoscopy or Cologuard), and a further component captures dates and their relationships to clinical terms of interest (from the text “The patient had a screening colonoscopy along with a resection of a hyperplastic polyp on January 22, 2020” it extracts the relation Colonoscopy: 1/22/20).
When we process data sequentially like this, each component carries its own risk for errors, and those errors add up. And each is dependent on the accuracy and errors of earlier components in the sequence. Initial errors can be magnified by later components. It’s like a Telephone game — one person whispers the message to the next, but as errors accumulate, the final output can diverge markedly from the original message. When you start with poor data quality, the errors produced by your NLP system will multiply rapidly. Garbage In. Garbage Out.
How Poor Data Quality Affects Your Results
The good news is that there has been a lot of progress in technologies such as optical character recognition (OCR) as well as language models that are more robust to certain data quality problems. The bad news is that poor data quality and missing metadata are still significant problems that limit the overall impact and value you will get from your NLP solutions.
Common Data Quality Problems that Impact Your NLP Results
What data quality problems impact NLP the most? Here are the three big issues we commonly see.
1. Faxes and OCR. Data derived through Optical Character Recognition (OCR) from images, including faxes, are one of the most common sources of poor NLP recognition and accuracy. OCR has two separate impacts on downstream NLP accuracy. First, OCR produces misspellings (for example “angina” might turn into “anpina”) which can be hard to recognize and adjust for in the components that map terms to concepts. Second, OCR mangles certain tables, lists, and other data structures that provide a lot of meaning about relationships embedded in the structure. Mangled tables and lists are a much bigger problem when you are using NLP for HEDIS and Quality as opposed to Risk Adjustment because Quality often requires extraction of relationships embedded in these structures.
2. Lack of Encounter Metadata. Chased charts meant for manual review are often missing crucial metadata that is not as important to human reviewers. For example, we may lose information about what type of document is represented (e.g., whether a specific note is an outpatient or inpatient visit, or whether it is a particular specialty encounter). This information is critical to processing the data. It’s also critical to know whether the evidence the system finds is allowed, per the HEDIS specification. Worse, sometimes the data is concatenated in such a way that NLP can’t tell where one encounter ends, and another begins. All these issues typically require more advanced methods to deal with missing metadata.
3. Differences among Electronic Health Record Systems. Subtle differences in how EHRs store text can introduce anomalies (like random formatting tags or unwrapping of tables). These subtle differences are often unique to a specific EHR, and sometimes even to a specific version of an EHR. It’s critical that your NLP vendor has pre-processing tools to account for and clean up these differences. Without this added level of expertise, you could lose valuable information downstream.
Solutions
Fortunately, there is A LOT that your organization can do to limit data quality problems and maximize the impact of the technology – not just in one silo, but across your entire organization.
Pick an Interoperability Partner
For Health Plans that are using NLP for RA and Quality, the most important investment you can make is selecting an interoperability partner that can handle your data acquisition. Ideally, you’ll pick a partner that understands your downstream analytics needs and vendors. Astrata has been proud to partner with ELLKAY as our data integrator of choice. With their many years of experience and deep expertise in data integrations, we trust ELLKAY to get us the data exactly the way we need it. That helps us deliver maximum accuracy and value to our customers.
Go to the Source
Use data that is as close to the source as possible and focus on acquiring digital data as opposed to image-based data (such as a faxed chart). Digital data includes Digital PDFs, HL7 feeds, plain text files (and in some cases CCDs and CDAs). Here are two simple changes that can improve your results.
- If your providers download charts to document closed gaps, educate their staff to produce a digital PDF rather than faxing the chart.
- If you use a vendor to chase charts, modify your contract to acquire digital PDFs instead of image-based PDFs.
Make Sure your NLP Vendor has Deep Experience Managing and Processing Text
One of the secrets to picking an NLP vendor is to look for a team, not just a product. EHRs, Health Information Exchanges, and internal data warehouses constantly generate new data-quality challenges, whether it’s missing metadata that needs to be imputed, or strange new characters inserted by your provider’s EHR. A team — like ours at Astrata — with decades of experience processing data from a variety of systems, will also have models, tools, and techniques to catch and correct common and not-so-common data quality problems.
Above All, Think About Your Data from an Enterprise Perspective
The most important thing your organization can do is to think about data needs from an enterprise perspective. While one operational unit may be ingesting charts for manual review, and doesn’t mind low-quality faxed charts, another unit may need data on those same members, but in higher quality form for NLP and analytics. Getting out of your silos and talking to your leaders and colleagues about how to improve the overall data quality for ALL your business units can help you formulate a better and cheaper long-term data strategy.