March 29, 2022 | AI and NLP, Year Round Prospective HEDIS®
Why Your Risk Adjustment NLP Engine Won’t Cut It For Quality Measurement
One NLP engine to rule them all? Astrata’s president explains why it’s not that simple, how an NLP engine optimized for Risk Adjustment can let Quality gaps slip through the system, and why we need robust new technologies that can make Quality an equal player in improving population health.
Last month, I wrote about some basic measurement topics to help bring us all up to speed on how NLP is validated. This month, I’m going to use those same principles to illustrate why your Risk Adjustment NLP engine, which you know and love, is going to break your heart when applied to Quality measurement.
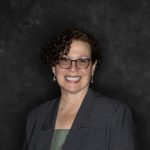
Rebecca Jacobson, MD, MS, FACMI
Co-Founder, CEO, and President
Please Don’t Throw Anything at Me Yet.
Let me just start by saying that everyone who hears me say this wants to throw tomatoes at me. And I get why. It would be great if one NLP engine could solve both problems. We know Risk Adjustment and Quality are two very important and closely intertwined activities in most healthcare organizations. More on that towards the end of the blog.
The key to understanding why one NLP engine won’t serve both purposes goes back to the tasks and goals associated with these two fundamental health plan operations.
The Risk Adjustment Task. When the task you are doing is Risk Adjustment, the goal is to find all cases where HCC (ICD) codes should have been used and apply them. Each HCC code is associated with a specific dollar amount. And the health plan team is attempting to optimize for HCC coding and associated HCC revenue. It isn’t so important if the coders must look at a few more false positives. The value of each case that can be correctly labeled usually far exceeds the labor costs of those extra false positives.
The Quality Measurement Task. When the task you are doing is Quality Measurement, and particularly year-round, prospective quality measurement, the goal is to reduce the human effort it would take to review an entire population to determine the measure status of each and every member. Ideally, we would like to automate this process one day. Whether a human being checks every case (the current state) or the NLP system makes the decision (a desired future state), we need to be sure that we are only reporting measures as compliant if they truly are compliant.
Remember back to our discussion about Precision and Recall last month? Pop quiz: Which metric would you want to try to optimize for each of these two tasks above?
That’s right – for Risk Adjustment, you are trying to optimize for Recall. But for Year-Round Quality Measurement, you are trying to optimize for Precision.
And that, in a nutshell, is why your NLP engine for risk adjustment is going to break your heart when you try to use it for Quality. I know you want to consolidate vendors but trust me, this isn’t the place to do it. Go get yourself an NLP engine that was built for Quality.
Stepping off my soapbox now. Let’s dig a little deeper and understand some of the nuances.
It’s hard to have it both ways
What is it about clinical NLP systems that frequently puts us in the position of needing to choose between Recall or Precision? It’s another long and complicated question suitable for its own blog, but to simplify a little: one of the most common reasons is poor data quality and lack of metadata. In a world of access to ample, high-quality, electronic data and metadata, I suspect our systems and models would eventually reach both high precision and high recall.
The problem is that our current clinical data environment doesn’t fit that description at all. And we are constantly trying to code our way around that. We may need to perform optical character recognition (OCR) on scanned images or try to automatically classify document or encounter types because we don’t have the metadata. When we do this, we introduce errors that can get magnified with subsequent pipeline components. Then we have a choice: We can either suppress the problematic data, which leads to higher precision but lower recall, or we can let it pass through, which leads to higher recall and lower precision. Depending on what we are doing (like Risk Adjustment versus Quality) we have to pick our poison. Unfortunately, without better data, it’s hard to have it both ways.
There are a few other things that Risk Adjustment engines don’t have that are critical for Quality, and one of those things is temporal information extraction. Specifically, a Quality NLP engine needs to extract valid dates of service and link them to the event with high accuracy. The state of the art has been moving quickly in this area and seems ready for commercial tech, but it’s still a bit of an art form to make these temporal linkages.
Do No Harm
OK now back to our story about Risk Adjustment and Quality Measurement. In fact, there is something even more fundamentally different between Risk Adjustment and Quality that is critical to this decision analysis, and it has to do with the asymmetric costs of false negatives and false positives in Quality Measurement when compared with Risk Adjustment.
Let’s start with Risk Adjustment. If my NLP system presents an additional HCC code that is not truly present (a false positive), I know that the medical coder can simply reject it. The cost of the medical coder reviewing those additional false positives can be weighed against the cost of missing HCC codes that would give a more accurate picture of the burden of disease in the population, and therefore produce more accurate risk adjustment to reimbursement. We can see that there is a tradeoff between false positives and false negatives, as discussed above. Importantly, we are trading off dollars for dollars.
Now let’s look at Quality. As you probably know, “closing a quality gap” can mean two very different things. First, it can mean documenting a closed gap in cases where members are compliant based on the narrative, but there is no associated claim. Second, it can mean properly intervening in a member with a true gap. And it’s this second group that we really care most about – because these are the patients who could develop a colorectal or cervical cancer without proper interval screening, or end up with sequelae of chronic diseases like diabetic nephropathy because they did not receive appropriate and timely therapy.
If we are trying to get to the desired state of quality measurement automation using NLP, we have to recognize that false positives have clinical costs in the form of reduced opportunities to intervene. That’s why we want to be sure that we don’t record non-compliant members as compliant, minimizing the False Positives, even if it comes at the cost of some extra cases that need to be reviewed. It’s not just a financial calculation.
The takeaway – you need Precision for Prospective HEDIS. It’s a better metric to optimize for, from both a business and clinical perspective.
Risk Adjustment and Quality – A Perfect Match?
So, what’s behind this desire to cook up Risk Adjustment and Quality together in a single piece of technology? One reason why I think people really want to do Risk Adjustment and Quality with a single NLP engine is that they are looking for a technology answer to a much more complex clinical and business question. A question we don’t have a good answer for yet. And that question can be framed like this:
How Do I Use Both Risk Adjustment and Quality Together to Improve the Health of My Population?
It’s a really good question and one that I am thinking about a lot lately. An analogy I often use is that Risk Adjustment is like a survey marker. It tells you exactly where your population is in terms of the burden of disease. But Quality is like a compass, it tells you where you need to go to move your population to a healthier state. Good population health means doing both Risk Adjustment and Quality really, really well. But it’s difficult to do either one of those things well without excellent technology. The scale and scope of working with populations is nothing like working with individuals.
When it comes to technology, Risk Adjustment has had a big head start. Having just returned from RISE National, I can say that the breadth and sophistication of NLP risk adjustment technology is truly impressive. Unfortunately, there has been so much less effort and investment in building out these kinds of technologies in Quality. And I think this is part of the reason that most people are looking for one platform that can do both. If only we could take all that cool technology in Risk Adjustment and make it work with Quality.
But I look at it differently. I think that to make Quality an equal partner to Risk Adjustment, we are going to need technologists to focus really deeply on the unique and specific needs of Quality, not try to simply repurpose a technology that was made for a different task. And that is what Astrata has set out to do – create the technology to help payers make that leap to Digital Quality so that they can marry their excellence in Risk Adjustment with excellence in Quality measurement and make a real difference to the health of their populations.